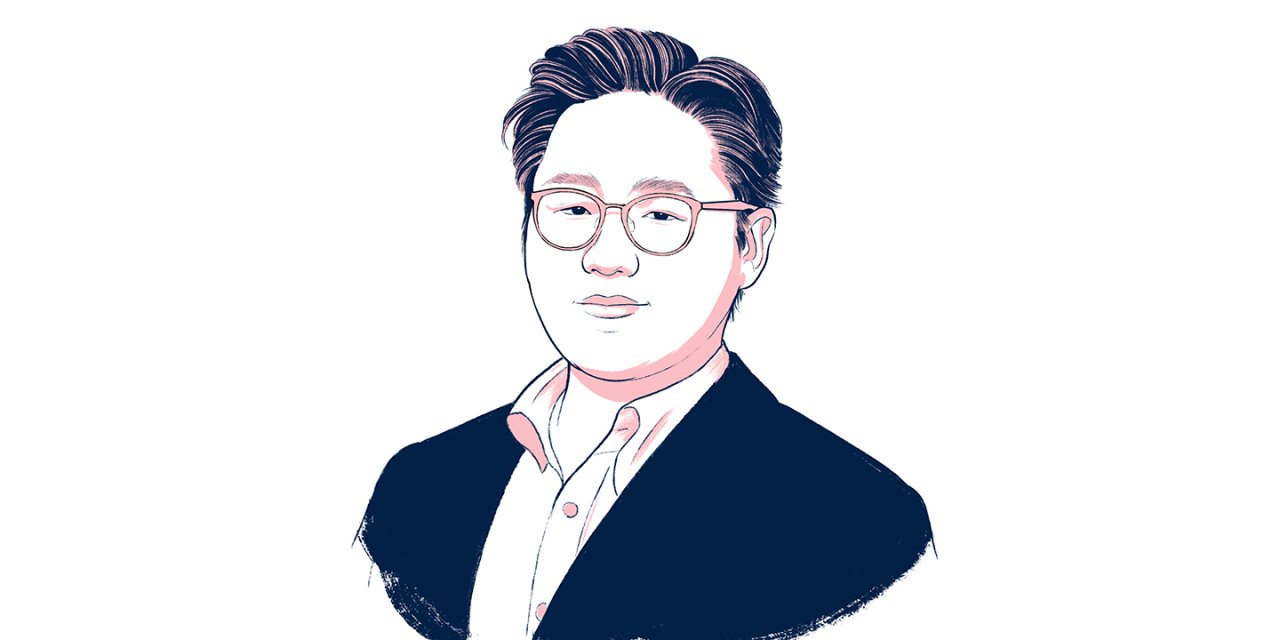
As scientists gain a deeper understanding of cancer, we have begun to tailor treatments to specific types of the disease. By putting a cancer tumor through advanced molecular testing, we can find out what proteins and genes are specific to this particular tumor and match it to the most effective treatment. The results are significant improvements in the survival rates of those patients fortunate enough to have a type of cancer for which a treatment is available.
The trouble is, too few patients are so fortunate. Despite a great deal of cancer research conducted over the past few decades, only a handful of targeted therapies have reached clinical practice--between 8-and-15 percent of cancer patients in the US are eligible to receive targeted therapy. We've made great strides in cancer treatment, but we need to do better.
Part of the problem is that cancer is a complex disease involving not only hundreds of genetic mutations, but other biological mechanisms as well. At present, we rely almost exclusively on genetic testing of cancer tumors to find a matching therapy. The results are mixed. For example, if a breast-cancer tumor tests positively for over-expression of the HER-2 gene, the drug trastuzumab will probably be an effective treatment; but if the tumor is present in the lung, that treatment won't work as well. Similarly, the existence of the BRAF V600E mutation in skin cancer tumors means that vemurafenib will probably be effective; but not in the case of colorectal cancer.
What goes missing in molecular analyses is relevant information about the type of tissue a cancer appears in. Whether a tumor arises in the breast or the lung or the colon is relevant to what the treatment options are for drugs that directly target a tumor. It may be even more important for immunotherapy treatments, in which drugs target the interaction between tumor cells and immune cells rather than tumor cells directly.
Artificial intelligence is giving us a more comprehensive view of the battle that rages in a tumor between cancer cells, drugs and the immune system. When human pathologists look at a tissue sample under a microscope, they disagree 48 percent of the time on the diagnosis. That makes it hard to develop a way of comprehensively analyzing tissue type. Now that tissue slides can be scanned and digitized, however, the power of AI software is being brought to bear on vast amounts of data unavailable to a human pathologist. By training AI algorithms on tissue-slide images of cancer patients and data on clinical outcomes in response to treatment, we can make predictions based on a wider scope of complexities than traditional, non-digital pathology can manage.
Ultimately, no single type of data would be sufficient to precisely predict a tumor's response to treatment. Some combination of data that includes genetics, tissue-type analysis and other factors will be necessary. Ultimately, we believe that AI-powered data-driven analysis will help grow the number of patients who can benefit from targeting cancer therapies.
Brandon B. Suh, M.D., M.P.H., M.B.A., is CEO of Lunit, an AI company that develops technology for making data-driven decisions in cancer care.
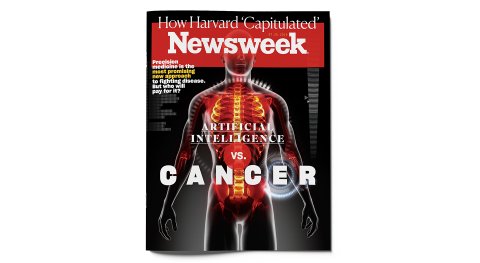